How to successfully implement a Machine Learning project
Digital transformation involves a huge change in the way we work and take advantage of technology. Machine Learning has become a priority for many companies: it is estimated that investment in Machine learning and Artificial Intelligence (AI) will go from 12,000 million dollars in 2017 to more than 57,600 in 2021 (according to a study by Oracle). And machine learning helps to make more intelligent and better decisions, with a level of security that would have been inconceivable a few years ago, in addition to increasingly automating tasks and business processes, streamlining these processes and increasing business efficiency.
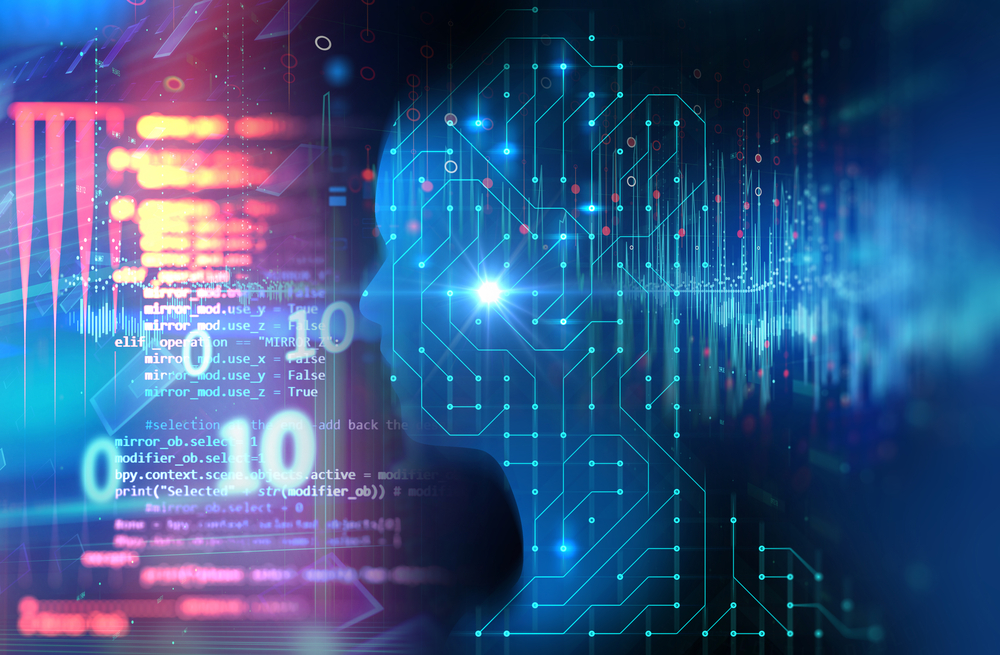
9 Keys to successfully implement machine learning in your company
Machine learning is a method of data analysis which automates the creation of analytical models. It is a discipline of artificial intelligence based on the concept that systems can learn from data, identify patterns and make decisions without human intervention (or with minimal intervention). By constantly producing data, machine learning solutions adapt independently and learn from both the new information and the previous processes.
Most companies who handle large amounts of data (Big Data) are recognising the value of machine learning (for example, the industrial, which obtains information from such different sources such as the internet of things, sensors, etc.).
While they already obtain a first added value thanks to analytical or Business Intelligence tools (for example, Tableau), with machine learning tools they advance even further in the use of their data to work more effectively and obtain significant competitive advantages.
If you are interested in getting the most out of your business data and automating processes like you never imagined you could, now is the time to apply a machine learning strategy in your organisation. To guarantee success in this process, at Intelligence Partner, we offer you 9 keys to successfully implement machine learning in your company:
- Machine learning also involves a cultural change. The technology associated with machine learning and algorithms evolves at great speed, and it isn’t easy to keep up with them. The main change must therefore be in the culture of the business: collaboration between the different business areas and the shared use of information must be encouraged so that the implementation of machine learning is successful.
- Define an objective as clearly and specifically as possible. It is important that the teams that are going to tackle machine learning projects identify the problems they want to address, applying the maximum degree of specification: for example, having the objective of increasing online sales by a specific percentage is not the same as specifying the wish to increase online sales by the desired percentage through monitoring visitors to the site.
- Make sure you have reliable data: the quality of the data is essential so that the machine learning tools can carry out their work effectively. If you opt for a supervised learning method, this source data must also be tagged so that the algorithm learns to predict the correct output tag (in this case, the company must have previously implemented a strategy of acquisition and tagging of solid and economically viable data. If you opt for a non-supervised learning method, it will not be necessary to have tagged data, but it must be 100% reliable.
- Rely on an integrated platform. The most profitable investment in a first machine learning project is the platform to carry it out on. It is highly recommendable to rely on one whose tools are fully integrated, such as Google Cloud Platform, instead of building an environment of applications from different manufacturers and whose integration capacity is yet to be proven. In the case of Google Cloud Platform, of great interest are its specific tools for undertaking machine learning projects.
- Start the project with a set of delimited data. The amount of information held by an organisation does not guarantee that all of this data is valid. When starting a Machine Learning project, it is much more desirable to focus on smaller sets of already valid data, since the cleaning process of the Big Data (in other words, its conversion to Small Data) is very long and expensive.
- Always look for simplicity. In English, we can use the expression “Keep IT simple”. Wherever possible, it is better to look for simplicity in the scope of any machine learning project than to build complex and expensive neural networks.
- Start with small projects. Both in terms of return on investment and gaining experience, it is highly recommended to start with small projects or those that cover very specific points of the business processes. This way, they will be executed and perfected until the team can start tackling larger machine learning projects, and you will discover other issues to solve with machine learning tools.
- Form multidisciplinary teams. If the project is only carried out by the Systems team, the efficiency of the machine learning project is reduced. Bringing together the different business areas involved in the processes affected contributes numerous nuances and adds fundamental considerations for the success of the project. These teams will decide the best way to reach the objective set through:
- Algorithms: useful to solve a class of new problems.
- Frameworks: use frameworks such as TensorFlow to create new models of machine learning and solve the unique problems of your business. Frameworks are the ideal point that offer you the greatest flexibility.
- API: take advantage of the API packages if they directly meet your needs without requiring any modification.
- View, verify and control versions. Data is a fundamental part of a machine learning project, and to manage this enormous volume of information, it is necessary to rely on tools such as those offered by Google Cloud Platform, like Google Cloud Dataprep and BigQuery, to view the data and verify the results in each phase of the process. It is also important to apply a versions control, to clearly identify the data to act upon and avoid costly misunderstandings.
At Intelligence Partner, as a Premier Partner of Google Cloud, we have significant expertise in Google Cloud Platform and in its Machine Learning capabilities.
If you want to undertake a machine learning project in your organisation, contact us and tell us about your situation. Our experts will analyse it in depth and will offer you a detailed response, 100% adapted to your needs.